- Infrasync Technology Services
- Posts
- Infrasync Newsletter #17 -Don’t Lose Control - Practical Implementation of AI for Water Utilities
Infrasync Newsletter #17 -Don’t Lose Control - Practical Implementation of AI for Water Utilities
Moving Towards AI

Three Step AI Process
More and more utilities are working to improve their data and AI capabilities. This is mostly driven by a need from utilities to do more with less. They have older systems, less funding, and fewer workers than ever before. This is not focused on replacing workers but rather giving them the tools and resources for today’s challenges. With each new technology innovation there is also a risk of failure because of rushed implementation.
Thankfully, institutional IT companies such as Nvidia, Microsoft, Amazon Web Services, and OpenAI are making it safe and easy for water utilities to implement new AI systems.
Once you already know what your drivers are and have detailed out how to achieve those through the use of AI then the implementation can be straightforward. The general process for implementing AI solutions can be simplified down to a 3 step process: Prep, Analysis, Implementation.
Step 1 – Prep
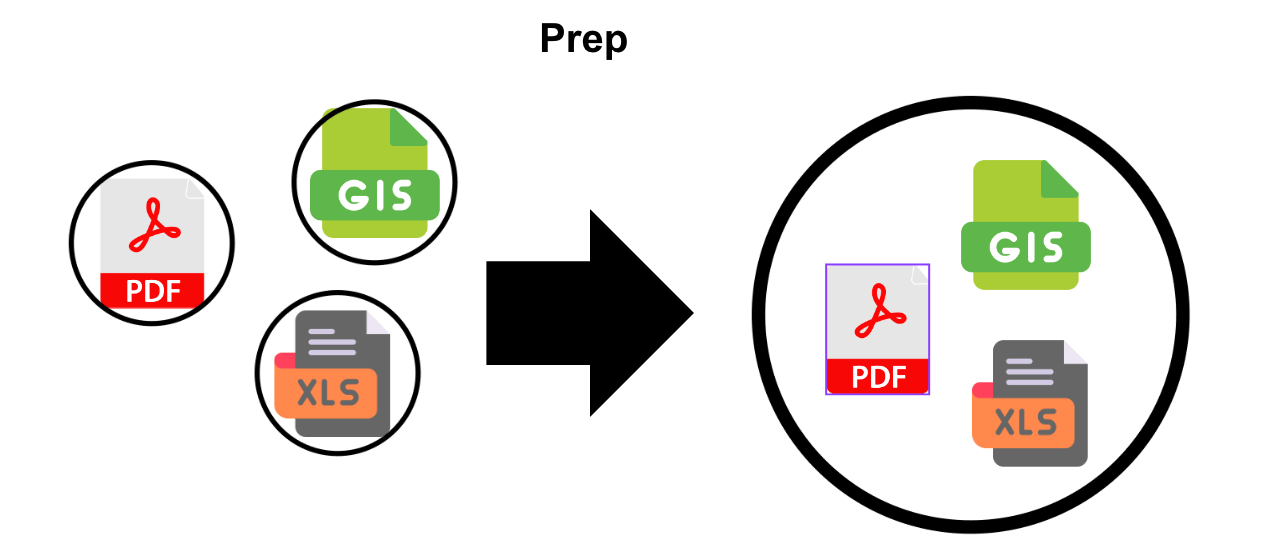
Prepare all your existing data for use by the AI system. Typically, this means bringing your GIS, SCADA, and other data systems together. For example, if you want to use AI to help find water quality issues before they get out of control then the following data would be needed:
· GIS – Location of water lines, valves, connectivity, and diameters.
· SCADA – Water produced, the quality, and timing
· Work Orders – Historical water quality issues and reports, also any flushing activity.
· Hydraulic Model – Typically in EPAnet format to estimate the flows and pressures around the system
· Meter Data – Used to get water demand in near real time
If all your data from the above sources check the boxes of accurate, relational, and relevant then it will make connecting the data together trustworthy. There are many example of utilities that were negligent in one of the above areas and when it came time for action they were left to manually figure it out instead of leveraging AI.
This greatly depends on the exact type of AI you need to implement. Machine Learning models are different than Large Language Models (LLMS). The LLMs that are rising in popularity such as OpenAI need the data structured in a certain way. This is the “vectorizing” of the data. This means that instead of a well ordered rows and columns like you would expected to see in an excel spreadsheet or a relational database this more closely mirrors the human mind in organizing and recalling information.
A simplified visual is above to show how similar words, concepts, and ideas get grouped together. This means that if you have a water distribution issues then it’s closely associated with anything to do with pressure, water quality, pipelines, etc but it likely won’t make many connections to other things in the utility such as pump maintenance or conference visits.
Step 2 – Analysis

This is the actual results of the AI typically used to save time, money, or improve safety, or maybe even all three. There are many types of AI analysis that we covered in an earlier blog post it may be worth a revisit.
Predictive Models - AI predictive models are decision-making tools that help identify patterns, trends, and relationships in their data.
Machine Learning - Machine learning is the ability of machines to learn and improve from experience without being explicitly programmed to do so.
Generative AI or Large Language Models – This approach uses deep learning techniques to generate natural language text that is similar to human writing.
Step 3 – Implementation
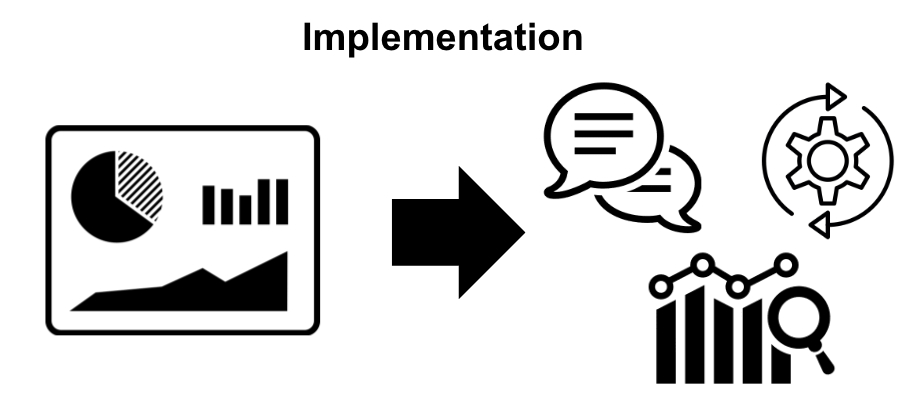
This is what each project is aiming for, the results and the useful part. The analysis and results must be brought back into the everyday usage of the utility. The results and outputs only matter if actually used.
Common integrations examples include:
- Predict pipeline breaks – based on previous failures and your pipeline materials, age, pressures, and other factors you can predict with 90%+ confidence which pipelines will fail. This is typically run once a year and used for capital project planning. Integrations include GIS and work order management.
- Predict sanitary sewer overflows – Based on historical overflows plus current weather predictions, this gives you up to 80% confidence in what sewer manhole will overflow, when, and how much. Then you can mobilize ahead of time to reduce the impact. Or given enough time in advance even clean out the lines and bring in by pass pumping. This type of analysis is typically running every few hours and as frequent as every 15 minutes during rain events. Integrations include GIS, Hydraulic Models, Manhole Sensors, and Weather Data.
- Predict treatment effluent – Based on historical influents, treatment conditions, and current operations you can predict with about 90% confidence what the discharge quality will be based on what you have coming in. This allows the operations team to ramp up (or down) chemical dozing, air blowers, side stream processes, and more. This type of analysis is typically running 24/7 and updating every 5 minutes or so. Integrations include SCADA and Weather Data.
- Respond to Public Inquiries – Based on utility records and standard procedures this is typically an online chatbot to help customers find out how to pay their bill, current water conditions, or when to go for specific help. More and more utilities are implementing this into their websites and mobile applications so that customers can get their information quicker. Integrations include Billing, Customer Accounts, and Web Services.
What should water utilities do?
If you haven’t engaged your IT department and ask them how ready for AI integration are you? You may be closer than you think.
If you have Microsoft sharepoint and database they are actively pushing more OpenAI integrations. Low hanging fruit includes taking all your training manuals and regulations and making those into a chatbot. This allows you to upload project files, documents, drawings and ask for an AI review instead of a manual page by page review.
A common roadblock is bringing in the SCADA information in a secure way. There are both physical and virtual one-way only devices and configurations to safely get SCADA information out of your on premise devices and into a database.
Finally, if you don’t already maybe form an AI working group across operations, planning, engineering, and other departments. It’s very common for AI driven projects to benefit multiple departments and so the costs can be shared across.
Additionally you may already have existing vendors and software providers with new AI capabilities since you purchased the equipment. It’s worth checking in with them to see what the new options are. Infrasync is water technology agnostic but we have seen some great innovations ranging from startups to the large corporate providers such as Microsoft.
Join the majority of water utilities and take a technology driven approach for today’s challenges!
Know someone who could benefit from this info? Forward this newsletter to them!
Make sense to connect?
Are you working to help your utility or technology company take the next step forward? If you want to talk through a challenge or share something interesting your team did please shoot me a note at [email protected] or schedule a 15 minute call here.